Artificial Intelligence: A Feature, Not a Benefit
AI is more than just a buzzword—it's a tool that can add substantial value when used right. This article shows you how to measure AI's impact. Remember, it will enhance a good product but won't save a bad one.
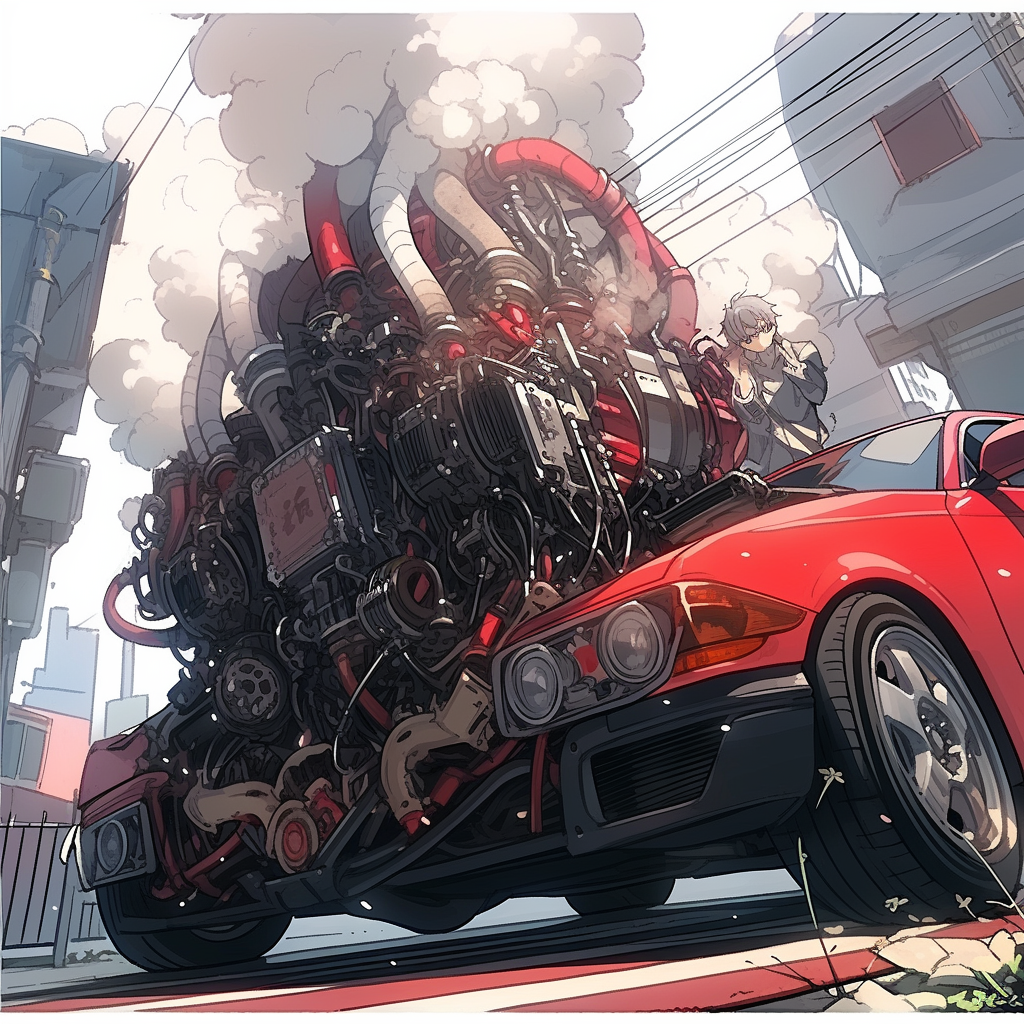
The allure of Artificial Intelligence (AI) in product development is undeniable. It's a buzzword instantly grabbing attention, promising a futuristic world where your product can learn, adapt, and evolve according to your customers' needs. There's a pervasive feeling that adding AI to any product is a surefire way to tackle pressing issues and boost engagement. Lately, though, it seems like AI fatigue is beginning to set in. In a world where everything is AI-enabled, distinction is lost, value is diluted, and customers yearn for the originality their products had before AI consumed the value proposition. A new mantra I’ve been using lately is unless you’re an AI-first company, AI is a feature, not a benefit.
Aligning AI With User Needs and Value Proposition
Don’t get me wrong. Integrating AI into your product or platform is an impressive feature. It opens the door to functionalities that may not be achievable without machine learning. AI can automate tasks, process large amounts of data quickly, provide predictive analytics, personalize user experiences, and much more. But these capabilities represent features, not direct benefits. They describe what your product does, not necessarily the value it brings to the customer or how it makes their life better.
Let's use Etsy as an example. Say Etsy integrates an AI-driven feature into its platform to automate and predict shopping preferences. The goal is to analyze users' purchases and browsing history and create personalized product recommendations for future shopping experiences.
While this AI feature is impressive and likely an upgrade to their current content recommendation system, it may conflict with Etsy's mission statement, "Keep Commerce Human.” Etsy strives to be a sanctuary for unique, handcrafted, and vintage items, where the journey of discovering and purchasing products can be as significant as the products themselves. Excessive intervention in this process may dilute the human aspect, the cornerstone of Etsy's appeal. This AI feature doesn't do much to enhance the core benefit of Etsy—providing a platform for human-centric commerce and discovery. A feature more in line with Etsy's mission would be personalized product suggestions curated by real people, such as staff members, experienced sellers, and shop owners from where the customer has previously made purchases. AI is a tool, and like any tool, its effectiveness depends on how it is used and how well it aligns with the mission and values of your organization.
With all the buzz, companies might fall into the trap of seeing AI as a magic solution for a product struggling to attract or retain customers. The reality, however, is that merely integrating AI into a product won't solve fundamental challenges. Issues like unclear product value, poor market fit, and user experience difficulties will persist. AI is a tool to enhance what's already working, to add value to a product that already resonates with its audience and aligns with their needs. In this context, AI is not a benefit but a feature. Bolting AI onto a misaligned product is akin to attaching a high-powered engine to a car with faulty steering - you're still gonna crash.
Developing an AI-first product is not an easy path to success. The marketplace for AI-driven solutions is already crowded and highly competitive. It takes more than just the AI label to stand out. The key lies in how AI can be harnessed to benefit the end-users, addressing specific pain points that non-AI products can't.
Measuring AI Value: Beyond Qualitative Data
But what does it look like when AI truly enhances the core benefit of a product or platform? The process begins with gathering qualitative data (See Table 1 below) about your customers' needs and desires. This can be accomplished through surveys, interviews, and user testing. However, qualitative data is the starting point in understanding whether AI adds value to your product. To fully grasp its impact, we must design and implement quantitative measures that gauge the effectiveness and accuracy of AI-driven features.
Method | Type | Description |
---|---|---|
Open-ended Surveys | Surveys | Enables free-form responses, offering insights into users' thoughts and experiences. |
Semi-Structured Interviews | Interviews | Follows a structure with predefined questions but allows flexibility to probe further based on responses. |
Usability Testing | User Testing | Observational method that identifies usability issues and improvements by watching users interact with a product. |
Think-Aloud Protocol | User Testing | Users verbalize their thoughts while using a product, offering insights into their thought process. It is a well-established protocol in usability testing and cognitive psychology for understanding problem-solving processes. |
Card Sorting | User Testing | Involves users grouping and categorizing items, which aids in developing intuitive navigation and information architecture. Its effectiveness has been demonstrated in numerous user experience (UX) and psychology studies. |
First Click Testing | User Testing | Measures which part of the design or interface the user interacts with first, providing insights into the user's initial behavior and if it aligns with the product's design intent. |
Table 1. Overview of Widely Used Qualitative Research Methods in User Experience (UX) Research
Consider a platform like Spotify, whose primary benefit is its ability to provide personalized music discovery. AI enhances this core benefit by analyzing user listening habits, understanding patterns and preferences, and making customized song recommendations. AI is employed not as a flashy add-on but as a tool that amplifies the product's core benefit, making it even more valuable to its users.
Implementing Direct Feedback Loops and Relevancy Scoring
Basic user metrics such as logins, session durations, retention, and Net Promoter Score (NPS) can offer a snapshot of user engagement. Still, they might not tell the whole story about the value added by AI features. This is where direct feedback loops and relevancy scoring become invaluable.
Direct feedback loops can be embedded into the workflow of AI-generated suggestions. We can garner valuable insights about the AI feature's performance by tracking how users interact with these suggestions. For instance, if your AI feature is a music recommendation engine like Spotify, user interface design could facilitate user feedback on each song recommendation, indicating the benefit level.
Relevancy scoring is another method that involves scoring the output of your AI – for example, a product recommendation – and tracking whether users engage more with higher or lower-scored recommendations. Monitoring this can help determine if a correlation exists between recommendation quality and user behavior.
Data-Driven AI Improvements
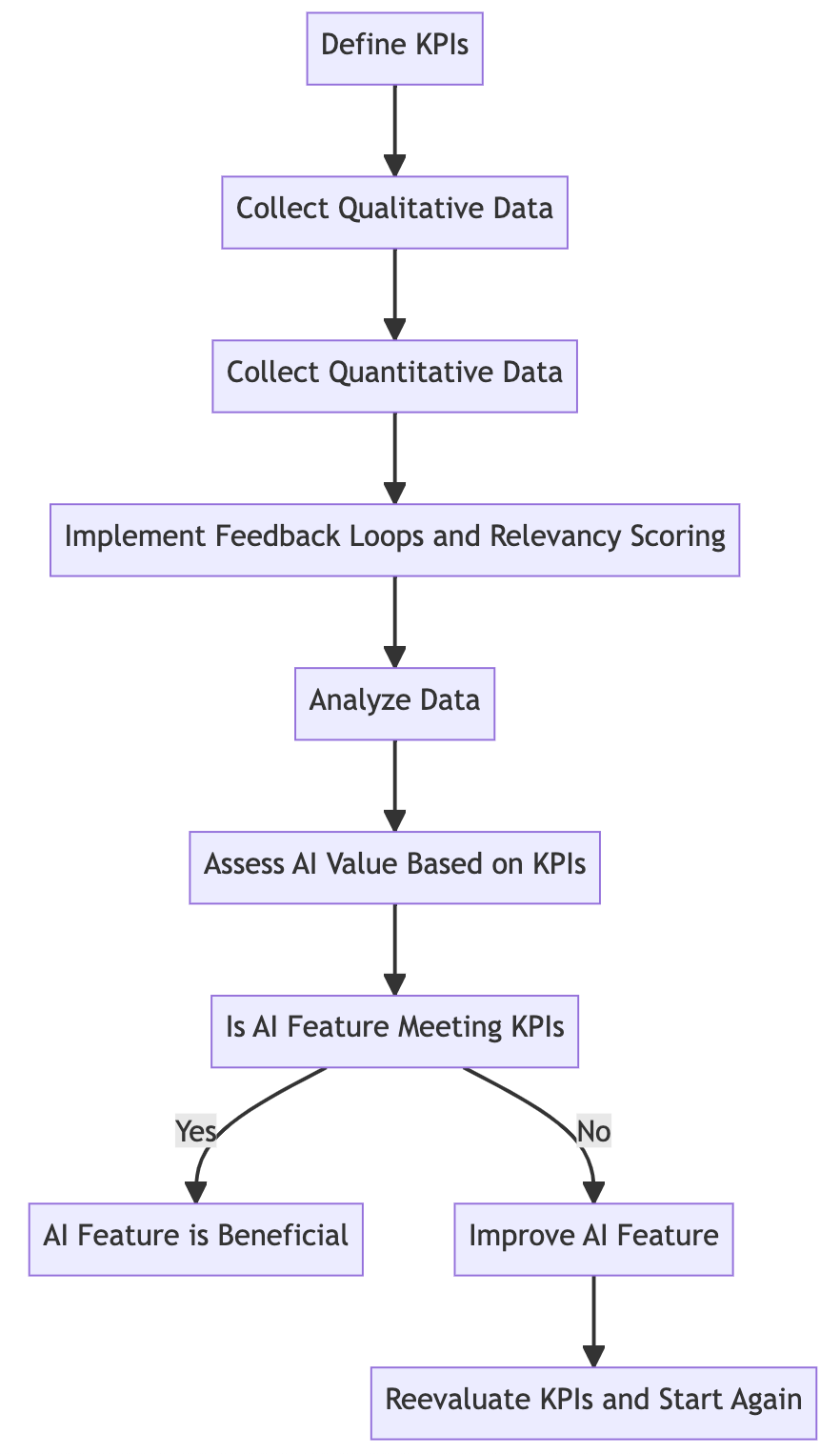
Gathering data isn't enough; the real value lies in how you use this data to adapt and enhance your AI features. The regular analysis allows for data-driven decisions and iterative improvements to your AI models and features, ensuring they align with user needs and the overall product value proposition.
Consider implementing A/B testing with and without AI features. This can isolate the AI impact, helping to assess whether AI features are driving engagement or causing unnecessary friction. The key lies in a systematic, data-driven approach to iteratively improve and refine your AI features based on direct user feedback and behavior.
AI as a Tool for Enhanced User Experience
While AI holds tremendous potential, it is still just a feature—not an automatic benefit. It doesn't eliminate the need for a strong understanding of your customers, their needs, and how your product can fulfill them. If a disconnect exists, no amount of AI will bridge that gap. Just as a high-powered engine won't fix a car with faulty steering, AI can't rescue a product that fails to meet its users' needs.
Understanding your company’s features and the emotional connection they create with your customers is essential for business success. Members receive exclusive access to the 12 meticulously designed prompts to give you deeper insights into your customer’s desires, expectations, and problems.